Why AI bias matters in safety reporting
by Dr Andrée Bates, Founder, Eularis and Dr Andrew Rut, CEO & Founder, MyMeds&Me
Safety reporting is an essential aspect of clinical research. In addition to providing valuable information to researchers and pharmaceutical companies, safety reporting to regulatory bodies like the FDA (United States) and the MHRA (United Kingdom) ensures both medicines undergoing clinical trials and those on the market adhere to agreed-upon standards of pharmacovigilance.
To be effective, reporting must be made simple and accessible to users and patients, and result in well-structured, granular data for researchers and regulators. Advances in technology, and especially in voice recognition and chatbots, have helped bring these goals closer. They offer a familiar alternative to traditional forms, with a more natural, conversational experience, without sacrificing on data.
The artificial intelligence (AI) that powers these solutions, however, is only as good as the data used to train it. And unfortunately, that data often carries biases that reduce the overall effectiveness of these otherwise very promising new approaches. So how can firms train AI to avoid bias in safety reporting, and what are the real-world risks of failing to do so?
AI bias: definition and examples
AI bias refers to the reproduction of social, economic, and other demographic biases in the analysis and output of data by an AI algorithm. There are a number of ways such biases can be introduced, including programmer bias, where a developer’s own biases are somehow reflected in the coding of the algorithm itself, and training bias, when the data used to “teach” an algorithm includes biases unaccounted for further downstream.
A particularly salient example comes from the unsettling discovery by the American Civil Liberties Union (ACLU) of racial biases inherent in Amazon’s publicly available and widely used facial recognition software, Rekognition. The ACLU reportedly used the software to compare 25,000 arrest photos against photos of then members of the US House and Senate. The results included 28 false matches, which were “disproportionately of people of color, including six members of the Congressional Black Caucus, among them civil rights legend Rep. John Lewis.”
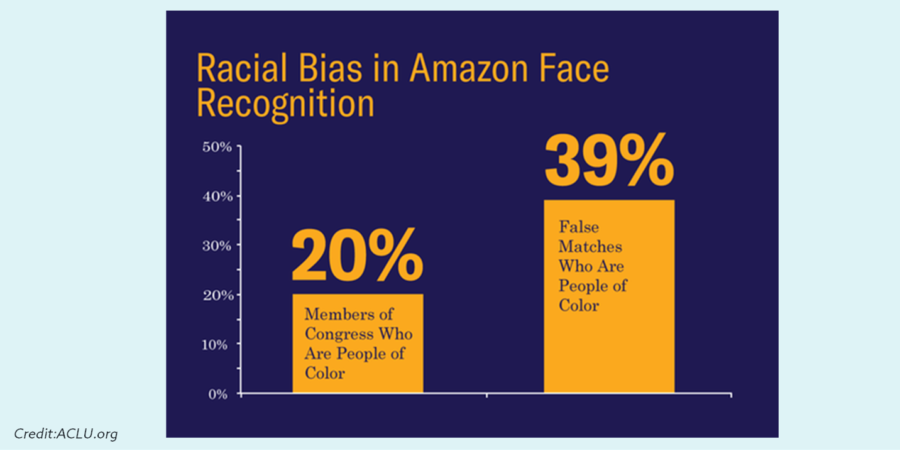
Speech recognition (SR) software is equally at risk. Many SR solutions have been trained on large datasets of predominantly white, male voices. As a result, the experience of users outside this privileged demographic is significantly poorer. Users are repeatedly asked to reiterate or reformulate their statement or query, directed to the wrong department or respondent, or presented with incorrect or irrelevant information.
The accuracy of SR software also varies significantly by dialect and accent. Indian English accents, for example, are interpreted with 78% accuracy, while Scottish English is interpreted with 53% accuracy.
Consequences of AI bias for drug safety
The practical implications of the presence of racial, gender, and other demographic biases in algorithms used in healthcare and biotech are significant and the moral implications downright chilling.
In one widely publicized case, an algorithm used to assess the health needs of more than 200 million American patients was found to carry significant biases against Black patients. The algorithm failed to account for differences in the cost-to-treatment ratios used in its training, and thus the level of care between patients with similar symptoms and situations diverged according to the color of their skin.
These findings are all the more pertinent given that certain biases are known to exist in safety reporting. Reported adverse drug reactions, for example, are higher in women than in men. Indeed, an analysis of sex differences in adverse event reporting in cancer clinical trials found greater severity of both symptomatic AEs and hematologic AEs in women across multiple treatment modalities.
Whether this reflects a pharmacological and biological reality or a cultural phenomenon, the fact remains that women are more likely to report incidents than men. Add to this the fact that women are (still) more likely to play the role of caregiver, and there’s little doubt that algorithms managing intelligent reporting are likely to encounter a large number of female patients. And yet, as we’ve seen, speech recognition is biased towards male voices.
What this means is that the experience for a majority of patients using an AI speech-based platform for reporting adverse reactions is likely to be relatively poor. Not only does this impact the quality of data such platforms can glean from interactions with patients, practitioners, and researchers, it may also reinforce existing social biases, further compounding the problem. Women are underrepresented in AI training data, which results in fewer successful AI interactions with women in practice, leading to further underrepresentation, and so forth.
From a practical standpoint, what this means for drug researchers and manufacturers in terms of drug safety is less accurate and precise profiles for new and existing interventions. In the example above, if women are more likely to experience adverse drug reactions, but reporting systems are not designed around their needs then biases in datasets, and resultant biases in reporting, inevitably translate into biases in published pharmacological and drug safety profiles.
What’s worse, in each of the examples given above, biases were only discovered after the offending algorithm had been put into use and impacted the lives of countless users. Similarly, regulatory bodies and researchers may believe they have a true and full picture of adverse reactions — when in fact that picture is heavily distorted. Such inaccuracies can have important consequences downstream, as analyses and subsequent decisions are distorted if based on unreliable or skewed data. This leads to potential harm, non-adherence, and products that fail to deliver their therapeutic promise.
How to avoid AI bias in safety reporting
By now, it should be clear the important role accurate data and bias-aware algorithm development plays in ensuring the successful use of AI in safety reporting. But how can firms avoid AI bias when using such tools?
First, let’s be clear: rarely are businesses or individuals accused of intentionally introducing bias into decision-making algorithms. In most cases, we can assume such biases are introduced unintentionally. This doesn’t make them any less dangerous or insidious, but it does mean that combating the problem is, first and foremost, a matter of acknowledging its existence.
While only 15% of businesses in Appen’s 2020 State of AI and Machine Learning reported that data diversity, bias reduction, and global scale were “not important”, a mere 24% considered it mission-critical. In the middle exist a host of businesses who are aware of the need for data diversity and bias reduction, but unsure how to proceed.
The following guidelines can help firms avoid AI bias in safety reporting (and beyond):
- Ensure stakeholders are educated and aware
A variety of resources exists for business leaders, developers, and researchers, such as AI Now’s research and reports, geared to “ensure AI systems are accountable to communities and contexts in which they’re applied,” while the Fairness, Transparency, Privacy interest group of the Alan Turing Institute is focused on ensuring that “the decisions made by machines do not discriminate, are transparent, and preserve privacy.”
- When deploying AI, create or leverage existing resources to mitigate bias.
Third-party audits, real-world testing, and rigorous follow-up protocols can all help catch bias early. There also exist a variety of resources for developers and businesses outlining best practices, including Google’s Responsible AI Practices and IBM’s AI Fairness 360, a “comprehensive open-source toolkit of metrics to check for unwanted bias in datasets and machine learning models.”
- Consider adding humans into the loop.
Human-in-the-loop is an approach that “reframes an automation problem as a Human-Computer Interaction (HCI) design problem.” Such an approach can take a variety of forms, like using AI to augment human decision-making or defining a certain threshold for ambiguity above which human intervention is required.
- Context matters: define specific parameters for individual reporting objectives.
Bias tends to creep in when project parameters are unspecific. Training data should be geared to and representative of the product’s target population. For example, solutions developed for reporting on an osteoporosis drug should take into consideration the fact that women are more prone to develop the disease than men and more likely to experience fractures.
- Ensure investments reflect expectations and objectives.
Firms must be prepared to engage fully in producing, offering, and championing bias-aware, inclusive AI solutions. This means fostering a diverse community of stakeholders and investing in more accurate and more diverse data.
Conclusion
The potential for improved reporting experiences for patients and researchers thanks to AI is undeniably great. But the risks to stakeholders at all levels from AI bias remain significant, with important practical and moral implications. Firms can help combat AI bias by promoting education and the use of novel or purpose-made resources, privileging human-in-the-loop approaches, properly defining the scope and context of reporting initiatives, and investing in diversity, both among stakeholders and within data.
By developing robust, bias-neutral AI algorithms tailored to user role, pharmaceutical companies can provide safety reporting solutions that are more engaging for users and deliver more reliable, representative, and richer data. This results in better analysis and more accurate signal detection so that communication on medicines to patients can focus on providing information most relevant to them.
Right now, we lack data on the impact of medicines on women. Therefore, how can Pharma and the healthcare system possibly make good decisions on how medicines are used, their safety profiles and risk mitigations? It is time for a radical rethink in how technologies can capture meaningful information across gender, demographics, and languages to fuel robust analysis and accurate product profiles.